The most workload of University of Patras has been paid on developing a novel data-driven deep learning application technique to identify the short-circuit faults that occur in the active distribution grids. The penetration of renewables at the edge of the grids, does not solely provide benefits towards the fulfilment of decarbonization objectives but also creates reliability issues.
Therefore, our application strives to facilitate grid operators to ensure the uninterrupted electricity supply of the end-consumers and a fast and accurate response to fault events by leveraging the proliferation of high-resolution measurement devices in distribution networks and the advancements in AI and particularly deep learning. We call it FLITC (not a fancy acronym though), which stands for Fault Location Identification and Type Classification because it is capable of identifying the short-circuit fault location and to classify the fault type into 11 different categories. The developed models – the well-known convolutional neural networks in conjunction with wavelet transformation for optimal preprocessing- grasp the spatial and temporal characteristics of three-phase voltage and current time-series measurements, thereby increasing network visibility in real-time. Our team conducted a series of simulations using synthetic data, which we provided in an open-source repository. This is very good for research community because the lack of data was the biggest issue during the development phase, that replicated a wide range of fault occurrence scenarios with 11 different types. The results demonstrate the efficacy of the proposed method, reaching an accuracy of 91.4% for fault detection, 93.77% for correct branch identification, 94.93% for fault type classification, and an RMSE value of 2.45% for location calculation.
After the models finetuning, real data digested from FEVER pilots to validate our concept in a real environment (very cool but also challenging to bypass the boundaries of the lab and try to connect to external systems). Last but not least, this work has been part of an excellent collaboration between two PhD students and a postgraduate researcher, who was partially published in the Applied Energy Journal.
The following figure graphically illustrates the main contributions of our study in the context of WP3. Graphical abstract summarizing our application: main drivers, contribution, and results.
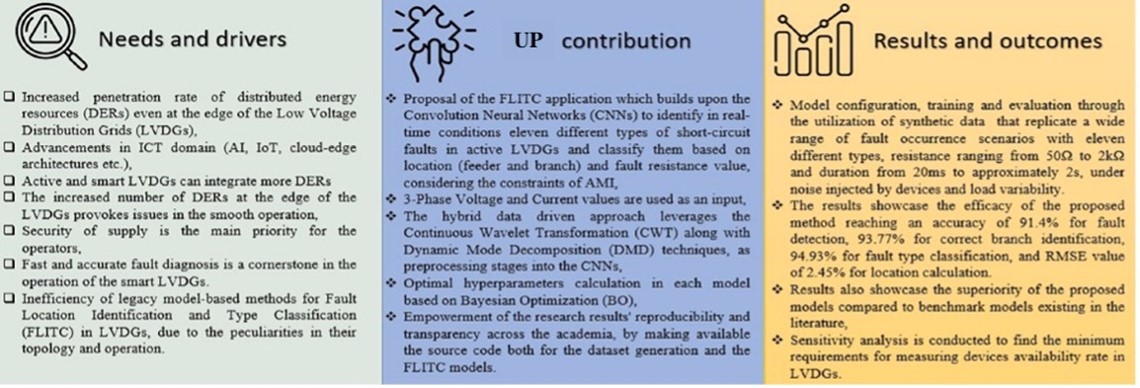
Preprint version of our paper in an open-source repository: https://www.researchgate.net/publication/369135226_Deep_Learning-based_Application_for_Fault_Location_Identification_and_Type_Classification_in_Active_Distribution_Grids